The evolution of the Security Operation Center(SOC)
Security Information and Event Management Platform (SIEM) have gradually become the center of SOC center. It creates a variety of interesting security detections(alerts) that security analysts have to respond to. Usually, a tier 1 analyst performs the initial triage of SIEM alerts and escalates high-priority ones to tier 2 or tier 3 for a more thorough threat hunting. The goal is to identify which actions the SOC team should take, how to stop a breach and how to prevent one in the future.
To assist the SOC team in quickly investigating and responding to security issues, new technologies are invented to assist security analysts such as Security Orchestration and Automation (SOAR). It is great to automate lots of low-level tasks and has great potentials but it also comes with a few challenges. First, security analysts have to know what to automate. Examples such as “if there are too many brute force logins, then lock down account” is not effective automation. Analysts feel risky to automate these responses because they lack confidence in the analysis.
The gap between Detection and Response
The gap between detection and response characterizes the manual effort required by security analysts to investigate each alert in order to either dismiss it as false positive or unveil the details of an attack to remediate it.
When a security alert arrives, a security analyst will ask…
- Is it real?
- What is the impact?
- How to clean up if it is real?
- How to prevent it if it is false positives?
- If it is worth automating it, how to automate it?
- Is it safe to automate?
Security teams are constantly facing these challenges. Unfortunately, their ability to review each alert and fully investigate potential threats is limited by the time-consuming effort that each alert requires. Consequently, security teams ignore early threat activity, only triaging high priority alerts which increases the risk of missing attacks.
The better response lies in better analysis.
Too many alerts and too little resources?
AI-based Security Analysis and Response:
DTonomy invented the solution to enable security analysts to reach an conclusion on false positive quicker and reduce the risk of missing true positives. How does it work? Let’s do a side by side comparison.
Without DTonomy
With DTonomy
Context Building:
step 1: rank security alerts by risk score
step 2: parse artifacts within security alerts
step 3: enrich security alerts with asset information from CMDB, external threat intelligence etc.
step 4: evaluate historical investigation on similar alerts (~20 minutes/alert)
step 5: manually correlate security alerts and create a case for them (~50 minutes/alert)
Context Building:
step 1: review correlated alerts in cases directly ranked by aggregated risk score
Decision:
step 6: if they are definitive true positives (1%), evaluate the impact via threat hunting and take actions to stop it.
step 7: if they are definitive false positives (40%), mark them as false positives.
step 8: if they are not clear at this moment(59%), conduct threat hunting(5 hours/alert) or leave them open and forget about it. (Huge risk!)
Decision:
step 2: if they are definitive true positive, assess the impact via threat hunting and take actions to stop it.
step 3: if they are definitive false positive patterns, resolve them at once!
step 4: for alerts that are uncertain, leave them there. DTonomy will pick them up if new relevant detection signals show up. (Reduce the risk of missing a true alert!) If nothing shows up, they will be in sleep mode until been waken up.
Optimize:
step 9: conduct group meetings to fine tune detection logic to avoid false positives(Risk of over tuning causing missing true positives!) , adjust risk of certain detections and package up some discoveries for other team to fix. (20 hours/week+)
Optimize:
step 5: DTonomy learns from your response continuously and automatically adjust risk score, identify false positive pattern which will be used to guide future investigations without touching master detections rules.(Reduce risk of tuning out important signals!)
Get to answer of “false positive” faster.
- When you identify security alerts as false positives, DTonomy learns patterns in your responses and continuously validates against more evidence. An example pattern could be detections from IP 2.3.4.5 are noticed to be false positive with 100% confidence.
- For incoming alerts, DTonomy’s pattern engine automatically identifies patterns among security alerts so you can identify offensive detection rules quickly. For example, a spiking number of alerts related to machine Machine_A show up within a short period of time. DTonomy AI engine enables you to spot this type of pattern quickly and conclude with root cause easier.
- Each case is ranked with an aggregated score from security alerts. The risk score of individual alert is updated intelligently when you resolve it as either true positive or false positive. So the risk score is totally personalized to your environment and gives you a more accurate estimation.
Reduce the risk of “false negative”.
- Even if certain alerts are mislabeled as false positives, do not worry. Our system will not filter those alerts. Instead, we continue to monitor them and connect them with new detections that may lead to strong evidence for true positive as a group of alerts.
Get a definitive answer on “false positive”.
- As news detections arrive, our pattern always looks back to historical alerts to see if they are connected within a pattern. If no new alerts link to an old pattern, all the alerts in the old pattern can be safely considered as false positives as they are not developing damages or stronger evidence on attacks.
Fit into your current investigation workflow
Instead of replacing existing threat detection capabilities and workflows, DTonomy has integrations with SIEM and SOAR platform so that it fits into your environment seamlessly.
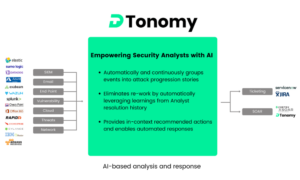